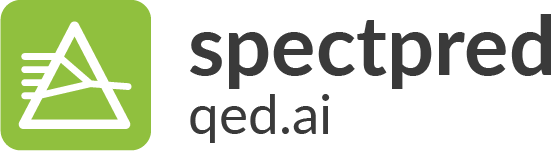
Predict soil properties from spectral measurements using the Bayesian Additive Regression Trees algorithm. After uploading your spectra, you can retrieve predictions of specified soil properties along with associated uncertainty estimates.
Launch Spectral Prediction AppBART Algorithm
The BART algorithm, devised by Robert McCulloch (University of Chicago) and his collaborators, has been shown to produce state-of-the-art predictions in many practical applications, while also providing natural statistical uncertainty estimates through a Bayesian framework. These properties can be compared with random forests and neural networks, which, while often exhibiting good performance in practice, do not provide rigorous uncertainty estimates. From a high-level perspective, the BART algorithm uses Markov Chain Monte Carlo algorithm to calculate the posterior distributions for each regression tree. The disadvantage of BART is the intense computation required for MCMC convergence. However, using centralized servers and cloud computation relieves local field labs of this burden by executing the training on high performance hardware, and then caching the results for other parties to use.
For more details about the BART algorithm, see the following documents:
Training Dataset and Prediction Results
The training dataset consists of 1831 paired wet chemistry and spectroscopy measurements of soil samples from across Africa, acquired during AfSIS Phase I which spanned from 2009 to 2013. The exact locations of these samples are displayed in the interactive map below, which you can pan and zoom into. Clicking on a pindrop reveals all wet chemistry measurements from that sample. The colors of the pindrops are binned into six different shades of green according to their total carbon percentage by weight, where darker shades of green correspond to higher percentages, and the number of samples in each bin is almost equal. Click on the icon in the upper-right corner of the app to open the map in a separate full-screen tab.
Note that all geospatial models are limited in their ability to be spatially extrapolated. When applying a model, the user must judge the density of the training dataset, and assess if new samples lie within sufficient proximity of the dataset that the model was trained on.
We compared the predictions for eight kinds of soil properties (C,N,Al,Ca,K,Mg,Na,pH) across three kinds of spectral machines (MIR, NIR, Alpha) with their true measured values. Clicking the button below will display a grid of interactive scatterplots that illustrate this comparison.
View Training Dataset Prediction Results